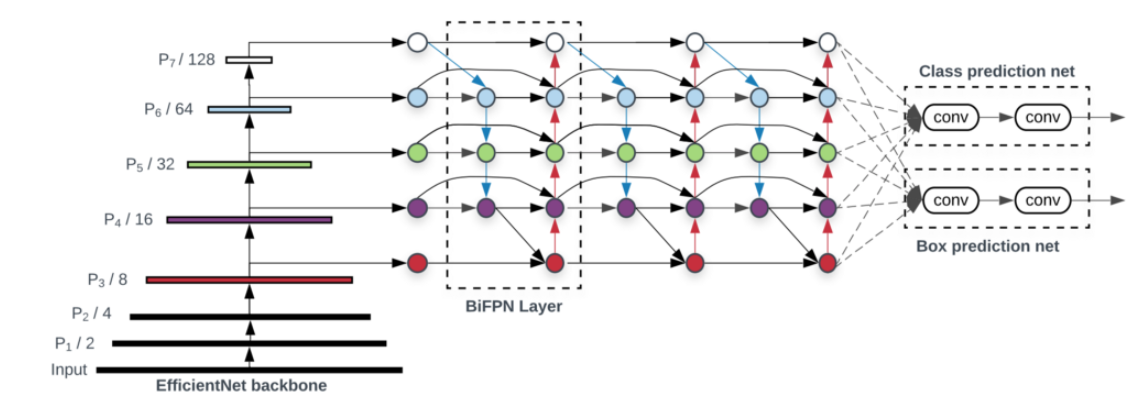
BiFPN (Bi-directional Feature Pyramid Network) 구조와 코드
2021, Oct 29
- 이번 글에서는
EfficientDet
에서 사용된BiFPN
구조에 대하여 살펴보도록 하겠습니다.EfficientDet
이 아닌BiFPN
만 살펴보는 이유는BiFPN
의 Feature 추출 효과가 좋아서 Detection 태스크가 아닌backbone
용도로BiFPN
이 사용되기 때문입니다. - 따라서 본 글에서는
EfficientNet
또는EfficientDet
에 관한 내용은 살펴보지 않고BiFPN
의 구조에 대해서만 퀵하게 살펴보도록 하겠습니다.
-
목차
BiFPN의 개념
BiFPN
은 기존에 많이 사용되었던FPN (Feature Pyramid Network)
의 개념에서 시작합니다.
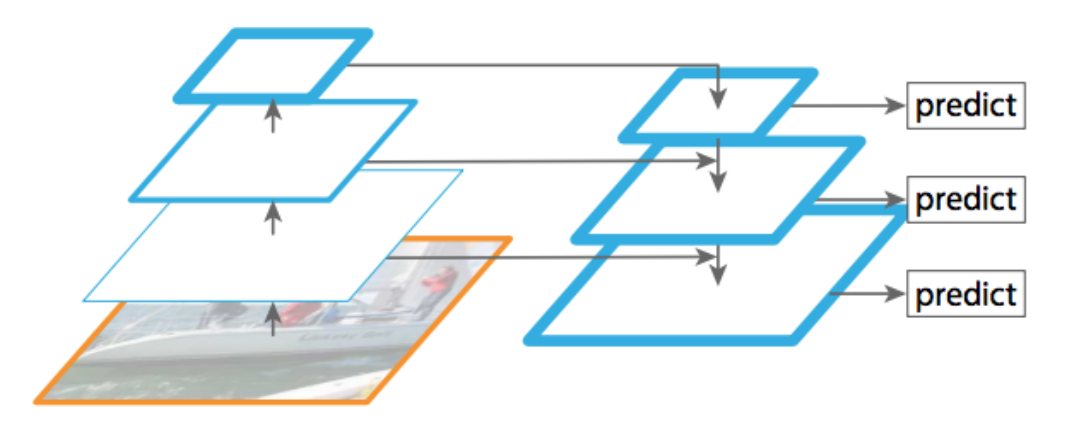
- 위 그림과 같은 형태의 feature의 피라미드 구조는 딥러닝 이전의 고전적인 컴퓨터 비전 연구에도 많이 사용되었고 딥러닝 기반의 컴퓨터 비전 모델이 개발되면서 기존의 지식을 모방하면서
FPN
구조를 가져와서 사용하곤 하였습니다. - 현재 까지도
FPN
구조는 Detection, Segmentation 등 다양한 컴퓨터 비전 태스크에서도 성능 개선을 위해 사용되고 있습니다.
FPN
구조의 핵심은 다양한 scale의 feature를 fusion하는 것에 있습니다. 이미지 상에서 feature를 찾을 때 서로 다른 크기의 객체가 존재하기 때문에 다양한 scale의 feature를 참조하는 것이 효과적입니다. 따라서 이 문제를 개선하기 위하여 예전부터 feature를 scale에 따라 피라미드 형태로 쌓아서 많이 사용하였습니다.- scale 변화로 축소된 feature에서는 넓은 범위에 걸쳐진 객체의 feature를 찾는 데 유리하고 큰 사이즈의 feature에서는 디테일한 객체의 feature를 찾는 데 유리한 측면도 있기 때문에 feature 간의 장단점이 있습니다.
- 하지만 고전 컴퓨터 비전에서는 다양한 scale의 피라미드를 모두 처리하는 데 계산 비용이 큰 문제로 인하여 지금처럼 효율적으로 사용하는 것에는 한계가 있었습니다.
- 하지만 CNN 구조에서는 layer간 feature를 fusion 하는 것이 매우 쉽고 계산 효율도 많이 증가하였기 때문에
FPN
을 효율적으로 잘 사용할 수 있는 장점이 있습니다. 따라서 feature를 fusion하는 것에는 큰 문제 없이 CNN에서 잘 사용하고 있습니다. BiFPN
에서는FPN
의 feature fusion 성능을 개선하기 위한 새로운 방식을 도입합니다.FPN
의 피라미드는 모든 feature map의 scale이 다르고 네트워크의 layer 깊이가 다르기 때문에 각 feature 별 의미의 차이가 크지만 단순히 더하는 방향으로 접근합니다.- 즉,
high resolution feature
는low-level의 의미
를 해석하고low resolution feature
는high-level의 의미
를 해석하는 것이 익히 알려져 있습니다.BiFPN
은 단순히 feature fusion을 위한 feature 피라미드의 단일 방향으로의 덧셈에서 더 나아가 양 방향 (bi-directional
)으로의feature fusion
방법을 제안합니다. - 즉,
lateral connection
을 이용한bottom-up
방향과top-down
방향으로의feature fusion
을 적용합니다.
BiFPN의 구조
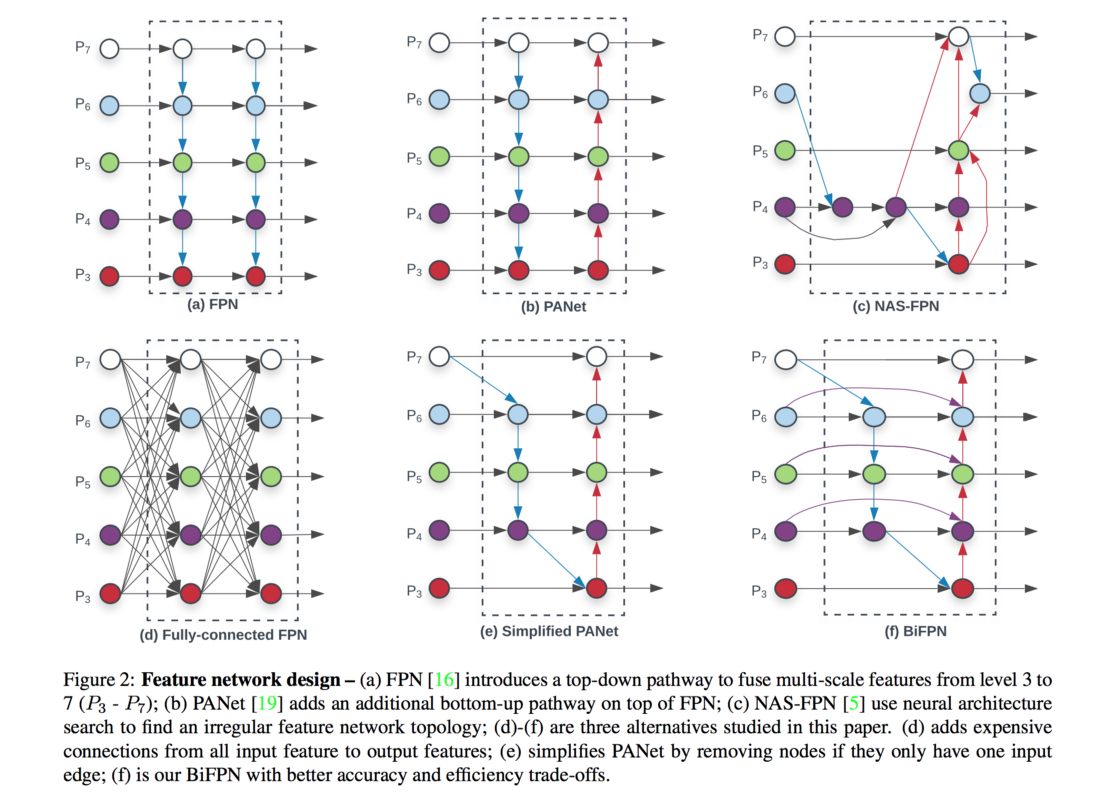
- 기존에도
FPN
의 구조를 개선하려는 시도는 많이 있었습니다. 위 그림과 같이FPN
을 기본으로 다양한 구조의FPN
이 제안 되었습니다.
- 기존
FPN
의 구조를 수식화 하면 다음과 같습니다.multi-scale feature
를top-down
방식으로 계속 합칩니다.
- \[P_{7}^{\text{out}} = \text{Conv}(P_{7}^{\text{in}})\]
- \[P_{6}^{\text{out}} = \text{Conv}(P_{6}^{\text{in}} + \text{Resize}(P_{7}^{\text{out}}))\]
- \[\cdots\]
- \[P_{3}^{\text{out}} = \text{Conv}(P_{3}^{\text{in}} + \text{Resize}(P_{4}^{\text{out}}))\]
- 위 식과 같은
top-down
방식만을 사용하는FPN
을 개선하기 위해 다양한 시도가 있었고 개선 방향의 핵심은 어떻게low-level feature
와high-level feature
를 합치는 것인가 입니다. - 다양한 시도 중
NAS
계열은 강화학습을 통하여 최적의 connection을 구하는 것이고 이것을 구하는 것 까지 새로운 학습을 해야하는 불편함이 있습니다. 또한 connection을 찾은 결과가 복잡하고 계산이 복잡할 수도 있는 단점이 있었습니다.따라서PANet
과 같은 형태로 단순하면서 효과가 좋은 connection 방법이 제시되었습니다.
- 아래는
BiFPN
구조를 얻기 위한 이력들 입니다. - 먼저
PANet
에서는 단 하나의 input edge만 있는 노드는 제거하였습니다. 각 node는 feature fusion의 역할을 해야 하는데 input edge가 하나만 있으면 feature fusion의 기능을 하지 못하므로 네트워크의 기여도가 줄어듭니다. 따라서 구조의 단순화를 위해 이러한 노드를 제거하여PANet
는 단순화된 구조를 가집니다. lateral connection
즉, 동일한 레벨에 있는 feature는 기존 input에 해당하는 feature에서 output 노드로의 edge를 추가합니다. 이처럼 동일한 레벨 사이의 추가 연산 없이 edge를 추가함으로써 계산 비용은 줄이고 성능 효과를 얻을 수 있습니다.PANet
과BiFPN
의 차이점 중 하나입니다.BiFPN
에서만 동일 레벨에서의 input feature를 output feature에 연결할 것을 확인할 수 있습니다.- 따라서 최종적으로 위 그림의 (f)번인
BiFPN
구조와 같이top-down
과bottom-up
구조를 동시에 사용하는bidirectional
구조를 사용하고 (f) 번의BiFPN
layer을 연속으로 누적하여 사용하면 여러 단계의BiFPN
layer를 쌓을 수 있습니다.
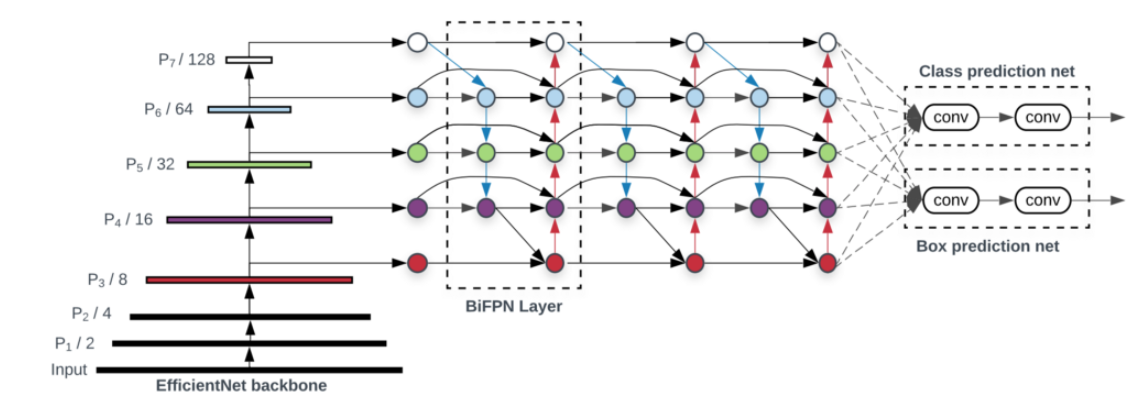
EfficientDet
에서BiFPN
을 적용한 구조는 위 그림과 같습니다.EfficientNet
의 Backbone을 그대로 사용하고BiFPN
을 이용하여feature fusion
을 적용합니다.- 점선 박스가 한 개의
BiFPN
의 layer이며 위 구조에서는BiFPN
layer를 3개 쌓인 것으로 생각하면 됩니다.
Pytorch 코드
- 아래 코드는
BiFPN
의 Pytorch 코드입니다. - 입력은 \(P_{3}, P_{4}, P_{5}\) feature 를 넣으면 \(P_{6}, P_{7}\) feature를 생성하고
BiFPN
layer를 거치게 됩니다. BiFPN
layer의 출력은 5개가 나오며 이 값은 각각 \(P_{3}, P_{4}, P_{5}, P_{6}, P_{7}\) 에 대응되는 결과 입니다.BiFPN
모듈의feature_size
는 출력 feature의 channel 수가 되며num_layers
파라미터를 통하여 몇 개의 layer를 쌓을 지 정할 수 있습니다.- 아래 입출력 예시는
feature_size = 64
이며 \(P_{3} \to P_{7}\) 로 거치면서 width, height의 크기는 1/2 씩 줄어들도록 설정하였습니다.
import torch
from bifpn import BiFPN
p3 = torch.rand(1, 8, 128, 128)
p4 = torch.rand(1, 16, 64, 64)
p5 = torch.rand(1, 32, 32, 32)
model = BiFPN([p3.shape[1], p4.shape[1], p5.shape[1]])
# outputs has 5 features
outputs = model([p3, p4, p5])
####################################################################
P3 : torch.Size([1, 8, 128, 128]) → torch.Size([1, 64, 128, 128])
P4 : torch.Size([1, 16, 64, 64]) → torch.Size([1, 64, 64, 64])
P5 : torch.Size([1, 32, 32, 32]) → torch.Size([1, 64, 32, 32])
P6 : → torch.Size([1, 64, 16, 16])
p7 : → torch.Size([1, 64, 8, 8])
####################################################################
BiFPN
코드는 아래와 같습니다.
import torch
import torch.nn as nn
import torch.nn.functional as F
from torch.autograd import Variable
class DepthwiseConvBlock(nn.Module):
"""
Depthwise seperable convolution.
"""
def __init__(self, in_channels, out_channels, kernel_size=1, stride=1, padding=0, dilation=1, freeze_bn=False):
super(DepthwiseConvBlock,self).__init__()
self.depthwise = nn.Conv2d(in_channels, in_channels, kernel_size, stride,
padding, dilation, groups=in_channels, bias=False)
self.pointwise = nn.Conv2d(in_channels, out_channels, kernel_size=1,
stride=1, padding=0, dilation=1, groups=1, bias=False)
self.bn = nn.BatchNorm2d(out_channels, momentum=0.9997, eps=4e-5)
self.act = nn.ReLU()
def forward(self, inputs):
x = self.depthwise(inputs)
x = self.pointwise(x)
x = self.bn(x)
return self.act(x)
class ConvBlock(nn.Module):
"""
Convolution block with Batch Normalization and ReLU activation.
"""
def __init__(self, in_channels, out_channels, kernel_size=1, stride=1, padding=0, dilation=1, freeze_bn=False):
super(ConvBlock,self).__init__()
self.conv = nn.Conv2d(in_channels, out_channels, kernel_size, stride=stride, padding=padding)
self.bn = nn.BatchNorm2d(out_channels, momentum=0.9997, eps=4e-5)
self.act = nn.ReLU()
def forward(self, inputs):
x = self.conv(inputs)
x = self.bn(x)
return self.act(x)
class BiFPNBlock(nn.Module):
"""
Bi-directional Feature Pyramid Network
"""
def __init__(self, feature_size=64, epsilon=0.0001):
super(BiFPNBlock, self).__init__()
self.epsilon = epsilon
self.p3_td = DepthwiseConvBlock(feature_size, feature_size)
self.p4_td = DepthwiseConvBlock(feature_size, feature_size)
self.p5_td = DepthwiseConvBlock(feature_size, feature_size)
self.p6_td = DepthwiseConvBlock(feature_size, feature_size)
self.p4_out = DepthwiseConvBlock(feature_size, feature_size)
self.p5_out = DepthwiseConvBlock(feature_size, feature_size)
self.p6_out = DepthwiseConvBlock(feature_size, feature_size)
self.p7_out = DepthwiseConvBlock(feature_size, feature_size)
# TODO: Init weights
self.w1 = nn.Parameter(torch.Tensor(2, 4))
self.w1_relu = nn.ReLU()
self.w2 = nn.Parameter(torch.Tensor(3, 4))
self.w2_relu = nn.ReLU()
def forward(self, inputs):
p3_x, p4_x, p5_x, p6_x, p7_x = inputs
# Calculate Top-Down Pathway
w1 = self.w1_relu(self.w1)
w1 /= torch.sum(w1, dim=0) + self.epsilon
w2 = self.w2_relu(self.w2)
w2 /= torch.sum(w2, dim=0) + self.epsilon
p7_td = p7_x
# print("p7_td : ", p7_td.shape)
# print("p6_x : ", p6_x.shape)
p6_td = self.p6_td(w1[0, 0] * p6_x + w1[1, 0] * F.interpolate(p7_td, scale_factor=2))
# print("p6_td : ", p6_td.shape)
# print("p5_x : ", p5_x.shape)
p5_td = self.p5_td(w1[0, 1] * p5_x + w1[1, 1] * F.interpolate(p6_td, scale_factor=2))
# print("p5_td : ", p5_td.shape)
# print("p4_x : ", p4_x.shape)
p4_td = self.p4_td(w1[0, 2] * p4_x + w1[1, 2] * F.interpolate(p5_td, scale_factor=2))
# print("p4_td : ", p4_td.shape)
# print("p3_x : ", p3_x.shape)
p3_td = self.p3_td(w1[0, 3] * p3_x + w1[1, 3] * F.interpolate(p4_td, scale_factor=2))
# Calculate Bottom-Up Pathway
p3_out = p3_td
p4_out = self.p4_out(w2[0, 0] * p4_x + w2[1, 0] * p4_td + w2[2, 0] * nn.Upsample(scale_factor=0.5)(p3_out))
p5_out = self.p5_out(w2[0, 1] * p5_x + w2[1, 1] * p5_td + w2[2, 1] * nn.Upsample(scale_factor=0.5)(p4_out))
p6_out = self.p6_out(w2[0, 2] * p6_x + w2[1, 2] * p6_td + w2[2, 2] * nn.Upsample(scale_factor=0.5)(p5_out))
p7_out = self.p7_out(w2[0, 3] * p7_x + w2[1, 3] * p7_td + w2[2, 3] * nn.Upsample(scale_factor=0.5)(p6_out))
return [p3_out, p4_out, p5_out, p6_out, p7_out]
class BiFPN(nn.Module):
def __init__(self, size, feature_size=64, num_layers=2, epsilon=0.0001):
super(BiFPN, self).__init__()
self.p3 = nn.Conv2d(size[0], feature_size, kernel_size=1, stride=1, padding=0)
self.p4 = nn.Conv2d(size[1], feature_size, kernel_size=1, stride=1, padding=0)
self.p5 = nn.Conv2d(size[2], feature_size, kernel_size=1, stride=1, padding=0)
# p6 is obtained via a 3x3 stride-2 conv on C5
self.p6 = nn.Conv2d(size[2], feature_size, kernel_size=3, stride=2, padding=1)
# p7 is computed by applying ReLU followed by a 3x3 stride-2 conv on p6
self.p7 = ConvBlock(feature_size, feature_size, kernel_size=3, stride=2, padding=1)
bifpns = []
for _ in range(num_layers):
bifpns.append(BiFPNBlock(feature_size))
self.bifpn = nn.Sequential(*bifpns)
def forward(self, inputs):
c3, c4, c5 = inputs
# Calculate the input column of BiFPN
p3_x = self.p3(c3)
p4_x = self.p4(c4)
p5_x = self.p5(c5)
p6_x = self.p6(c5)
p7_x = self.p7(p6_x)
# print(p3_x.shape, type(p3_x))
# print(p4_x.shape, type(p4_x))
# print(p5_x.shape, type(p5_x))
# print(p6_x.shape, type(p6_x))
# print(p7_x.shape, type(p7_x))
features = [p3_x, p4_x, p5_x, p6_x, p7_x]
# output has 5 features
output = self.bifpn(features)
return output
BiFPN Encoder 코드
- github 링크 : https://github.com/gaussian37/pytorch_deep_learning_models/blob/master/bifpn/encoder_bifpn.py
- 위 링크는 앞서 설명한
BiFPN
을 일부 경량화 한 후(B, C, H, W)
크기의 배치 단위의 이미지를 입력 받아서 FeedForward 할 수 있도록ResNet18 + BiFPN
형태로 구성한 코드입니다. - 코드를 일부 수정하여 encoder의 출력 사이즈를 변경할 수 있으며 decoder에서 활용하여 다양한 태스크에서 사용할 수 있습니다. (EfficientDet에서는 Object Detection에 활용한 것을 참조하시면 도움이 됩니다.)
x = torch.rand(1, 3, 512, 960)
encoder = Encoder(channels=64, num_layers=2)
outputs = encoder(x)
for o in outputs:
print(o.shape)
# torch.Size([1, 64, 64, 120])
# torch.Size([1, 64, 32, 60])
# torch.Size([1, 64, 16, 30])
# torch.Size([1, 64, 8, 15])