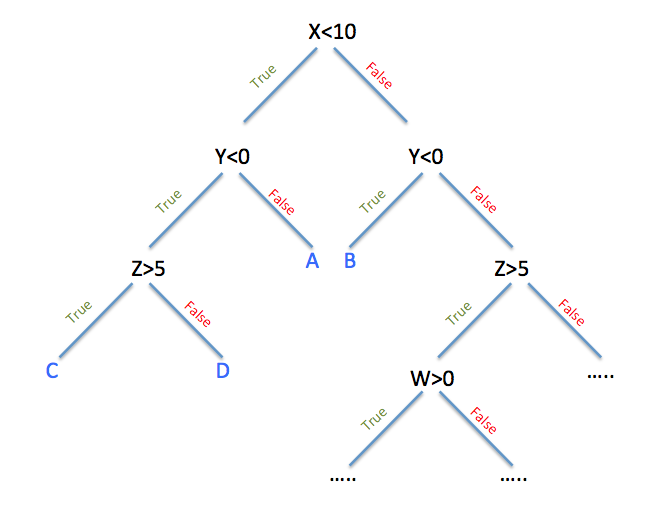
Decision Tree (Reference ML KAIST)
2018, Aug 28
Before dividing into Decision Tree, what is machine learning?
- A computer program is said that
- learn from experience E
- With respect to some class of tasks T
- And performance measure P, if its performance at tasks in T, as measured by P, improves with experience E
A Perfect World for Rule Based Learning
Imagine a perfect world with
- No observation errors, No inconsistent observations.
- (Training data is error-free, noise-free)
- No stochastic elements in the system we observe.
- (Target function is deterministic)
- Full information in the observations to regenerate the system
- (Target function is contained in hypotheses set)
Observation of the people
- Sky, Temp, Humid, Wind, Water, Forecast → EnjoySport
Sky | Temp | Humid | Wind | Water | Forecast | EnjoySport |
---|---|---|---|---|---|---|
Sunny | Warm | Normal | Strong | Warm | Same | Yes |
Sunny | Warm | High | Strong | Warm | Same | Yes |
Rainy | Cold | High | Strong | Warm | Change | No |
Sunny | Warm | High | Strong | Cool | Change | Yes |
Function Approximation
Machine learning is relative to applying function approximation well.
- Machine Learning ?
- The effort of producing a better approximate function
- In the perfect world of EnjoySport
- Instance
X
- Features : <Sunny, Warm, Normal, Strong, Warm, Same>
- Label : Yes
- Training dataset
D
- A collection of observations on the instance (Collection of Xs)
- Hypothesis
H
- Potentially possible function to turn X into Y
- h_i : <Sunny, Warm, ?, ?, ?, Same> → Yes
- Target function
C
- Unknown target function between the features and the label
- Our objective is expressing hypothesis
H
to target functionC
.
- Instance
Graphical Representation of Function Approximation
- x1 : <Sunny, Warm, Normal, Strong, Warm, Same>, h1 : <Sunny, ?, ?, ?, Warm, ?>
- x2 : <Sunny, Warm, Normal, Light, Warm, Same>, h2 : <Sunny, ?, ?, ?, Warm, Same>
-
x3 : <Sunny, Warm, Normal, Strong, Warm, Change>, h3 : <Sunny, ?, ?, Strong, Warm, ?>
- What would be the better function approximation?
- Generalization Vs. Specialization
- Instance x1 & Hypothesis h1 is
Generalization
- Instance x3 & Hypothesis h3 is
Specialization
Find-S Algorithm
- Find-S Algorithm
- Initialize h to the most specific in H
- For
instance
x in D- if x is positive
- For
feature
f in O- if \(f_{i}\) in h == \(f_{i}\) in x
- Do nothing
- else
- (\(f_{i}\) in h) ← (\(f_{i}\) in h) \(\cup\) (\(f_{i}\) in x)
- if \(f_{i}\) in h == \(f_{i}\) in x
- For
- if x is positive
- Return h
For example,
- Instances
- \(x_{1}\) : <Sunny, Warm, Normal, Strong, Warm, Same>
- \(x_{2}\) : <Sunny, Warm, Normal, Light, Warm, Same>
- \(x_{4}\) : <Sunny, Warm, Normal, Strong, Warm, Change>
- Hypothesis
- \(h_{0}\) = \(<\varnothing, \varnothing, \varnothing, \varnothing, \varnothing \varnothing>\)
- \(h_{1}\) = <Sunny, Warm, Normal, Strong, Warm, Same>
- \(h_{1,2}\) = <Sunny, Warm, Normal, Don’t care, Warm, Same>
- \(h_{1,2,4}\) = <Sunny, Warm, Normal, Don’t care, Warm, Don’t care>
- Any possible problems?
- Many possible hs, and can’t determine the coverage.
we live with noises
- We need a better learning method
- We need to have more robust methods given the noises
- We need to have more concise presentations of the hypotheses
- One alternative is a
decision tree
.
Good decision tree split the data as good ratio. Above example, A1 or A9 are features. In the case of A9, we have two options, True/False. If we choose True(left) then, we get correct answer 284/307. otherwise, 306/383, which is not good but not bad result.
In the decision tree, we should consider how to split the data in order to get good results.
Entropy
- Better attribute to check?
- Reducing the most uncertainty
- Then, how to measure the uncertainty of a feature variable
- Entropy of a random variable
- Features are random variables
Higher entropy
meansmore uncertainty
- \[H(x) = -\sum_{x}P(X=x)H(X=x)\]
- Conditional Entropy
- We are interested in the entropy of the class given a feature variable
- Need to introduce a given condition in the entropy
- \[H(Y|X) = \sum_{x}P(X = x)H(Y | X = x) = \sum_{x}P(X = x){-\sum_{Y}P(Y = y | X = x)log_{b}P(Y = y | X = x)}\]