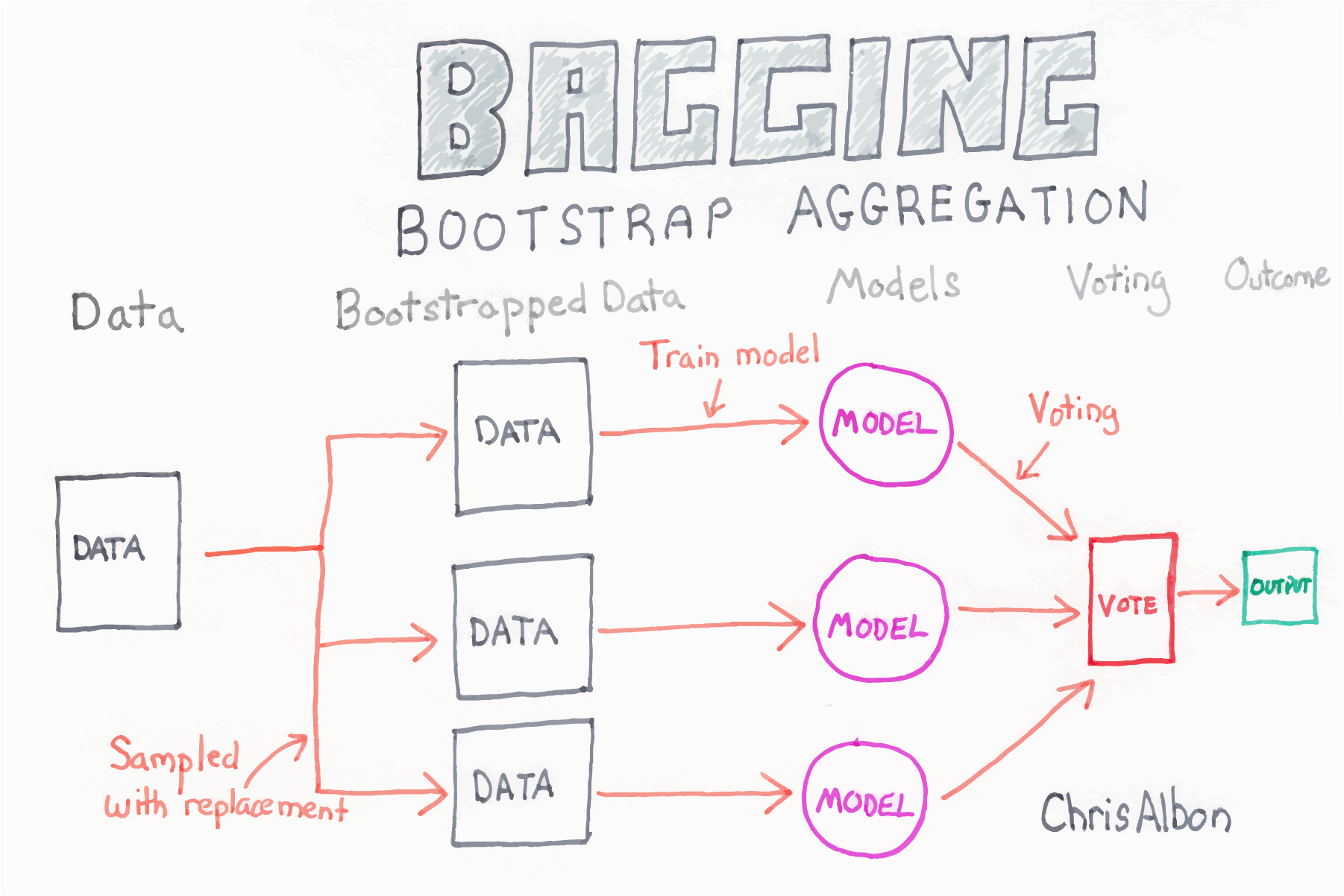
Overview Bagging
2018, Oct 02
All datasets are sampling of entire data. Let’s create various classifier with various sampling dataset. Also, with various sampling, we can make robust classifier.
As above, entire data can be separated to several samples. After ensemble of those, we can make robust classifer as third graph. Also, we call it that several weak classifier makes string classifier.
Sampling reduces overfitting problem. Even though results of samples make overfitting, Ensemble of those has good learning result. Or, sampling reduces variance.
What is the bootstrapping?
- sampling with replacement in learning dataset
- sampling n subset learning data
- bootstrapping means sampling without adding data
- .632 bootstrap
- when sampling d times, each datum is sampled with 0.632 probability.
- when sampling d times, each datum is sampled with 0.632 probability.
What is bagging?
- Bagging is Bootstrap Aggregation.
- Ensemble with n subsample
- Assign various data to one model.
- Proper to high variance model.(likely to get overfitting)
- Bagging is proper ensemble for models likely to getting overfitting well.
- Combination of each overfitting lessens this problem and works out.
- supports regressor, classifier
what is Out of bag error
- OOB is error estimation
- When using Bagging, validate the performance with not included data in the bag.
- It’s similar to deal with validation set
- Good standard for evaluating Bagging performance
How to use Bagging in sklearn
- base_estimator : One estimator
- Concept of bagging is using one classifier and many different data
- n_estimator : #bootstrap
- max_samples : data ratio to use
- max_features : feature ratio to use
BaggingRegressor is same with BaggingClassifier to use.
from sklearn.tree import DecisionTreeClassifier
from sklearn.ensemble import BaggingClassifier
clf = DecisionTreeClassifier(random_state=1)
eclf = BaggingClassifier(clf, oob_score=True)
cross_val_score(eclf, X, y, cv = 10).mean()